“Predicting better than pure guesswork, even if not accurately, delivers real value. A hazy view of what’s to come outperforms complete darkness by a landslide. The Prediction Effect: A little prediction goes a long way.” – Eric Siegel, Predictive Analytics: The Power to Predict Who Will Click, Buy, Lie, or Die
What is predictive analytics?
Predictive analytics is about using historical data, statistical algorithms, and machine learning to predict future outcomes. Spotting trends plays a role, but it’s mainly about quantifying what’s likely to happen next in order to take action ahead of time.
This is hugely useful for CMOs and marketing leaders because it helps you:
- Identify the leads that are more likely to convert
- Forecast the performance of your campaign before launch
- Predict churn risk and customer lifetime value
- Anticipate market demand and react accordingly
Predictive models analyze information like web behavior, purchase history, and social interactions, continuously refining data as more comes in – and making predictions more accurate over time.
Predictive vs. prescriptive analytics
It’s also important to mention prescriptive analytics – after predictive analytics tells you what might happen, this advises you on what to do about it. In short, prescriptive analytics recommends actions based on your data (such as historical data and real-time inputs).
It builds on predictive analytics but goes a step further by using optimization and simulation algorithms to suggest options.
On top of this, predictive analytics enables CMOs to forecast campaign results, customer behavior, and market shifts, whereas prescriptive analytics allows CMOs to better personalize strategies and drive performance.
Why predictive analytics is a CMO’s secret weapon
Smarter budget allocation
With marketing budgets increasingly under scrutiny, CMOs really need to prove the ROI of their efforts. Predictive analytics can help you do this more effectively, as it allows you to forecast where each dollar will have the most impact.
For instance, you may want to prioritize channels with the highest predicted conversion or engagement rate based on past performance and behavior patterns, instead of spreading your budget evenly across several channels.
Higher customer retention
Customer churn is expensive but, more often than not, it can be avoided. Predictive models can flag at-risk customers before they disengage, enabling targeted interventions like personalized offers, loyalty nudges, or re-engagement campaigns.
Hyper-personalization at scale
Predictive analytics leads to better personalization because you’re analyzing signals like browsing behavior, purchases, and content interaction. So, you can better tailor your messages to your customers automatically.
For instance, product recommendations before your customer even knows what they want, or content journeys that guide people seamlessly through the buying funnel.
Sales and marketing alignment
Predictive models allow sales teams to spend time on the right prospects (a.k.a., the ones most likely to close based on things like their past behavior).
This makes it easier to improve the handoff between sales and marketing, which, in turn, leads to better conversion rates.
Faster decision-making
Speed is crucial in marketing – you blink and the current trends have changed.
So, by using predictive analytics, you can get real-time insights that allow you to pivot campaigns instantly or tweak your existing campaign.
For example, a retailer could use it to determine the products that will be in demand next season, helping them to make better purchasing decisions.
Revenue growth
By targeting the right customer at the right time with the right message, you can drive more sales. Predictive analytics can identify high-value customers, cross-sell or upsell opportunities, and the best possible pricing.
Competitive advantage
Acting on insights before your competitors do can make all the difference. If you spot and act on trends early, you can innovate faster, adapt to changes quickly, and dominate market niches.
In fact, Salesforce’s Predictive Intelligence Benchmark Report says that predictive intelligence recommendations have been found to influence an average of 26.34% of total product orders, with the influence increasing to 34.71% after 36 months of implementation.
Customer segmentation and personalization
Predictive analytics can help CMOs and other marketing leaders serve more relevant content and offers to customers, allowing them to boost conversion and engagement rates.
For example, Netflix uses behavioral segmentation to recommend shows based on previous viewing habits, which helps them increase watch time and retention.
To implement this, you can:
- Integrate customer data from CRM, site behavior, and purchase history.
- Use clustering algorithms (e.g., k-means, hierarchical clustering) to group users.
- Sync segments with marketing automation tools (e.g., HubSpot, Salesforce).
Some of the KPI benchmarks you should consider are open and clickthrough rates, conversion rates by segment, and revenue per segment.
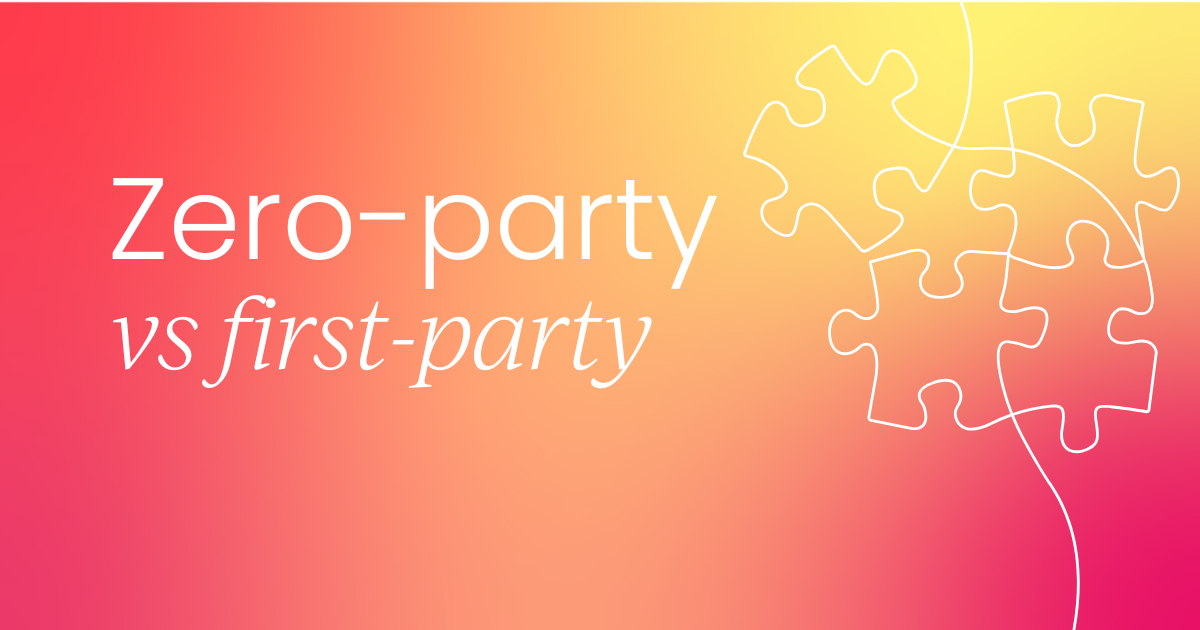
Customer lifetime value prediction
This helps you to identify and prioritize the highest-value customers for acquisition and retention.
Amazon, for instance, estimates customer lifetime value to decide where to offer free trials or next-day delivery. In essence, investing more in customers who will bring them more value over time.
So, you can use lifetime value insights in bid strategies on paid channels (such as Google Ads) and adjust your customer acquisition cost based on predicted customer lifetime value.
Churn prediction
This allows you to prevent or reduce revenue loss by proactively re-engaging at-risk customers.
A real-world example is Spotify monitoring drop-off in listening and login behavior – they send out emails and offers to boost retention before churn occurs.
Some KPIs to consider are churn rate before and after intervention, increase in your retention rate, and net revenue retention.
Campaign optimization
Maximize your campaign ROI by predicting what performs best in terms of audience, message, time, and channel.
Airbnb uses predictive analytics to determine the best timing and location for ad campaigns, which leads to increased bookings.
Forecast demand
The goal here is to anticipate spikes or drops in demand so that you can optimize inventory, staffing, and promotions.
Target predicts demand for products using sales data, holidays, and economic indicators (feeding into ad planning and supply chain).
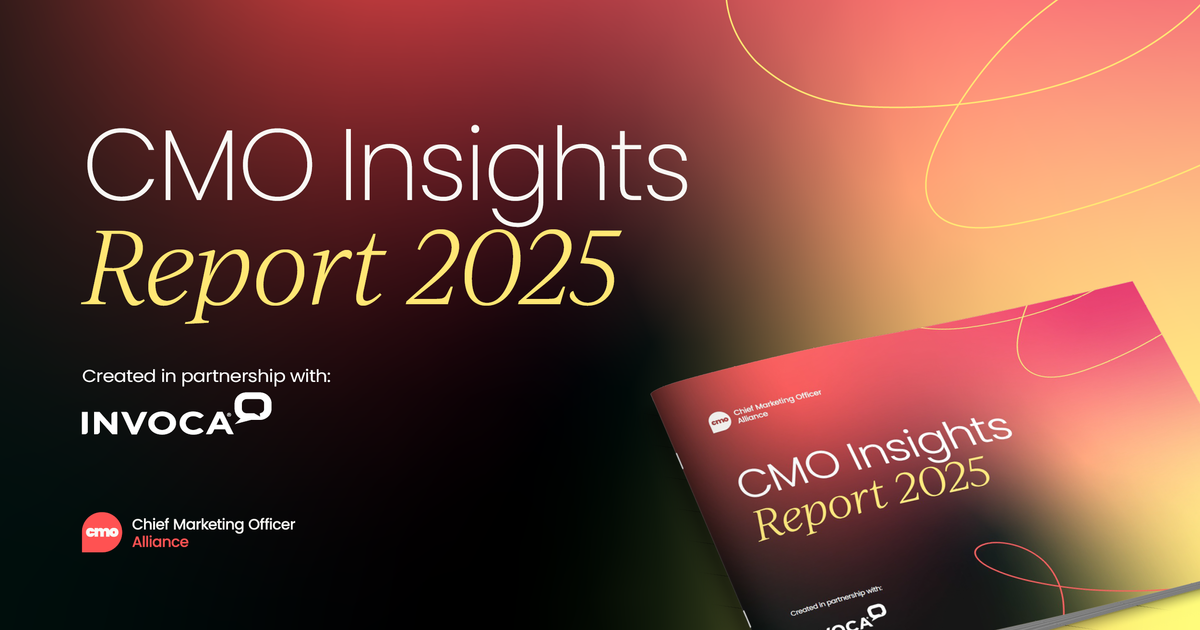
Better pricing strategy
You can predict optimal pricing to maximize your profit while also staying competitive.
Uber does this by using dynamic pricing algorithms that adjust in real time based on demand predictions and driver availability.
Optimize your media spend
This means predictive analytics allows you to allocate money across channels in a way that maximizes ROI on every dollar you spend.
Vodafone Italia implemented an AI-based co-pilot to enhance their digital advertising strategies. By combining cross-channel budget optimization with scenario simulation, Vodafone streamlined their budget planning process, leading to a 13% increase in revenue.
So, predictive analytics allows you to collect performance data across all your channels and adjust your budget dynamically.
Predict brand sentiment
If you can identify and address issues before they become a real problem (or even before they appear), you can protect your brand.
For instance, Nike analyzes social sentiment in real-time during product launches to spot potential backlash and adjust messaging.
You can also use AI to process reviews and support tickets, flag anomalies, and set up alerts that your team can reply to.
Create a better content strategy
After all, with predictive analytics, you can plan content that’s more likely to resonate with your audience, rank higher in SERPs, and convert.
HubSpot uses machine learning to predict which blog topics will generate leads and organizes content calendars accordingly.
This is something you can do as well. Analyze content performance by type, topic, format, and use AI to predict topic demand and the potential for engagement.
What is a downside of predictive analytics?
A key downside of predictive analytics is bias in the data. If the data used to train predictive models reflects societal biases, such as discrimination based on race, gender, or socioeconomic status, the predictions can reinforce that and learn to repeat them as well.
This can lead to unfairness, especially in things like hiring and recruiting.
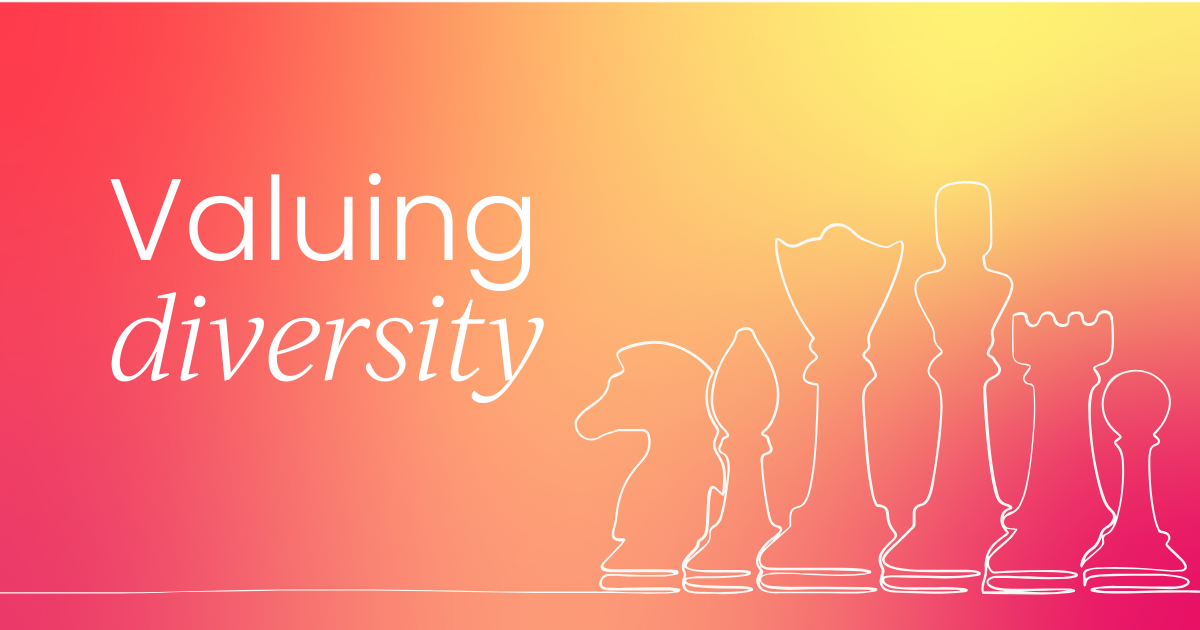
This is a huge issue because it can lead to unethical decisions, companies might face legal consequences if their practices are discriminatory, and people will lose trust (employees and customers alike).
Other downsides include:
- Data quality: Predictive analytics need to be trained on complete and accurate data, as otherwise it can create misleading predictions.
- Overfitting: This is when the model learns the “noise” in the data, details that aren’t relevant, instead of actual patterns. The result is that the model will struggle with new data because it never learned the real patterns.
- Black box nature: This refers to when the predictive model gives you a result (like a risk score or customer churn prediction) but doesn’t clearly explain why it gave that result.
More predictive analytics use cases
Leading healthcare institutions like the Mayo Clinic and the Cleveland Clinic use predictive analytics to improve their inventory management.
By employing AI tools, they can forecast supply shortages, streamline ordering processes, and optimize stock levels, which is crucial for reducing waste and ensuring critical supplies are available when needed.
The National Football League (NFL) employs predictive analytics to optimize its game schedules. By analyzing vast amounts of data, including team performance, travel logistics, and broadcast ratings, the NFL can generate schedules that maximize viewership and ensure fairness across the league.
“We’re starting to add a little bit more of the predictive analytics and the big data sets and really trying to make more science- and mathematics-based decisions while never completely removing the gut and feel.” – Mike North, VP of Broadcast Planning
WestRock integrates predictive analytics into its smart packaging solutions. Using AI and machine learning, they analyze supply chain data to forecast demand and optimize inventory levels.
This approach enhances productivity, reduces waste, and ensures timely delivery of packaging materials to clients.
In short
AI is making it easier for CMOs to use predictive analytics, and it’s fair to say that we’re moving towards a world where campaigns are optimized in real-time, messaging is always context-aware, and customer journeys are shaped by behavior and intent.
Struggling to prove the real impact of your marketing? You’re not alone.
CMOs are feeling the pressure, which is why we created The CMO’s Guide to Measurement, you’re go-to for AI-led strategies, smart triangulation, and better team collaboration.
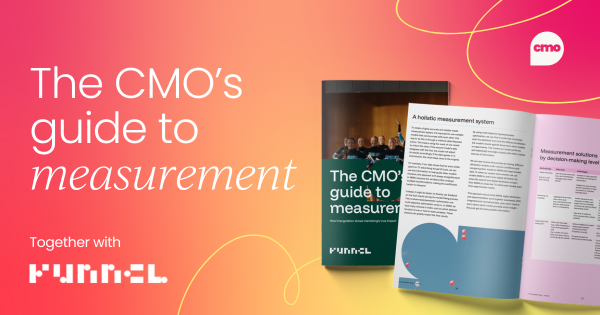